Nick Battaglia of Iowa is a financial industry professional who explains that in the fast-evolving landscape of finance, the integration of cutting-edge technologies has become paramount. In this article, Dominic Battaglia unravels the profound impact of machine learning on the financial sector, gaining valuable insights into its applications, challenges, and the transformative potential it holds for the future.
The financial world is continually evolving, and one of the most significant changes in recent years is the incorporation of machine learning into investment strategies. Machine learning, a subset of artificial intelligence, offers a powerful tool for financial professionals to make more informed decisions and enhance their investment processes.
In this article, Nick Battaglia of Iowa explores the fundamentals of machine learning in finance, key machine learning models, how they enhance investment processes, and the advantages and challenges of this transformation.
Nick Battaglia Discusses Machine Learning and How is It Used in Finance
Machine learning, a subset of
artificial intelligence (AI), focuses on developing algorithms capable of learning from data to make informed predictions. In finance, it involves using historical and real-time data to develop models that can predict market trends, analyze risks, and optimize investment strategies.
Nick Battaglia of Iowa explains that it can analyze market data, generate trading signals, optimize portfolios, and even assist in customer service through chatbots. It's an invaluable tool that enables financial professionals to leverage data for better decision-making.
Key Machine Learning Models for Investment Strategies
Various machine learning models are employed to analyze and interpret complex financial data, make predictions, and optimize investment decisions. Here are some key machine-learning models for investment strategies:
Regression Models
Regression models are used for predicting numerical values, making them essential for forecasting financial data like stock prices, interest rates, and asset prices. These models analyze historical data and identify patterns that can be used to make future predictions.
Classification Models
Dominic Battaglia of the University of Iowa says that classification models categorize data into distinct groups or classes, making them valuable for various applications in finance, such as stock prediction and fraud detection. These models analyze historical data and classify it into predefined categories, allowing investors to make decisions based on trends and patterns.
Reinforcement Learning Models
Reinforcement learning models are essential for algorithmic trading, where they learn and adapt to maximize rewards and minimize risks over time. These models use trial and error to learn optimal strategies for trading. They continuously improve their decisions by receiving feedback based on their past actions, making them suitable for high-frequency trading and portfolio optimization
Enhancing Investment Processes with Machine Learning
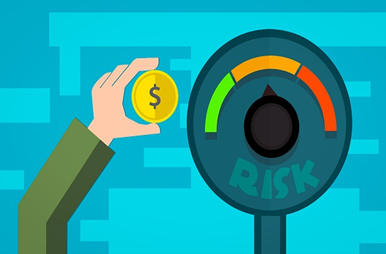
Machine learning offers a wide range of applications that can significantly enhance investment strategies. Here's how it is enhancing investment processes:
Stock Market Prediction
Machine learning algorithms, such as support vector machines (SVM), artificial neural networks (ANN), and long short-term memory (LSTM) networks, are widely used for stock market prediction. These algorithms analyze historical data, technical indicators, and other relevant market factors to predict stock price index movement, providing investors with valuable insights for their stock price prediction strategies.
By leveraging machine learning techniques, investors can:
- Identify patterns and trends that may not be easily detectable through traditional models
- Make better-informed decisions
- Sentiment analysis of news and social media can also be used to gauge market sentiment and anticipate price movements based on public perception and news events.
- Potentially increase their profits.
Risk Management and Asset Allocation
Nick Battaglia of Iowa explains that these models excel at identifying potential risks and optimizing asset allocation. By analyzing a broad spectrum of data, including market volatility, economic indicators, and historical performance, they can provide insights into how to minimize losses and maximize returns.
Moreover, it can be employed in investment management to:
- Accurately value and effectively manage assets, such as stocks and bonds
- Enable investors to make more informed decisions
- Ensure that investments are made in a way that maximizes returns while minimizing risks.
Algorithmic Trading
Algorithmic trading involves executing buy and sell orders based on pre-defined rules and strategies. Machine learning models can automate trading by continuously analyzing real-time market data and adjusting trading decisions accordingly.
Dominic Battaglia of the University of Iowa notes that high-frequency trading, where stocks are bought and sold in a matter of microseconds, is a prominent example of machine learning-driven algorithmic trading.
Advantages of Machine Learning
Machine learning offers a multitude of advantages across various domains, and its applications extend to numerous industries. In the context of finance and investment, it brings several key advantages:
Enhanced data analysis and speed
It can swiftly analyze vast datasets, providing insights that would be time-consuming or practically impossible for humans to process. This speed is crucial in financial markets where rapid decision-making is essential.
Improved accuracy and efficiency
These models can offer improved accuracy and efficiency in finance by automating complex processes and reducing the potential for human error. This can lead to more precise forecasts, better investment decisions, and more streamlined processes, ultimately resulting in lower costs and increased profits.
Adaptive learning and continuous improvement
Nick Battaglia of Iowa says that adaptive learning is a process in which machine learning algorithms are able to learn from their mistakes and adjust their parameters accordingly, thus becoming more accurate over time. This continuous improvement enables financial professionals to refine their investment strategies and adapt to changing market conditions, ultimately leading to greater success and profitability.
Expanding investment opportunities
Machine learning can identify new and previously unnoticed investment opportunities. By analyzing a wide range of data sources, it can uncover potential investments that might be missed by traditional methods.
Real-time market insights
Dominic Battaglia of the University of Iowa explains that this AI method provides real-time data analysis and insights which enables investors to react swiftly to market movements and make timely decisions that can be crucial in financial markets where every second counts.
Risk management and prevention
These models can detect and mitigate risks more effectively. By identifying potential issues and market anomalies, they help investors manage risks and minimize potential financial losses.
Unstructured and big data analysis
These models can handle unstructured data, such as text and images, and extract valuable insights from big data. This allows investors to consider a broader range of information when making investment decisions, including social media sentiment, news articles, and more.
Quantitative Analysis
Machine learning can handle complex mathematical and statistical analyses, making it invaluable for quantitative finance, risk modeling, and statistical arbitrage strategies.
Limitations and Challenges
While machine learning offers significant potential for enhancing investment strategies in the financial world, it also presents challenges and limitations that must be addressed in order to fully realize its benefits. Nick Battaglia of Iowa discusses the key challenges and limitations of it in finance.
Data quality
ML models heavily depend on the quality of data they are trained on. Inaccurate, incomplete, or biased data can lead to flawed predictions and investment decisions.
Risk of bias and discrimination
Bias and discrimination in machine learning can arise when algorithms are trained on data that is not representative of the population, leading to inaccurate results that can be used to discriminate against certain groups.
To reduce the risk of bias and discrimination in machine learning, financial professionals can:
- Utilize datasets that are representative of the population
- Employ transparent and explainable algorithms
- Implement techniques such as fairness-aware learning
Computing power costs
Nick Battaglia of Iowa says that the cost of computing power can be a significant consideration when implementing these models in finance, as the algorithms used to make decisions often require substantial computing resources. Factors such as the type of computer used and the cost of electricity can influence computing power costs, making it essential for financial professionals to carefully evaluate these factors when implementing machine learning models in their investment strategies.
Preparing for a Machine Learning-Driven Future in Finance
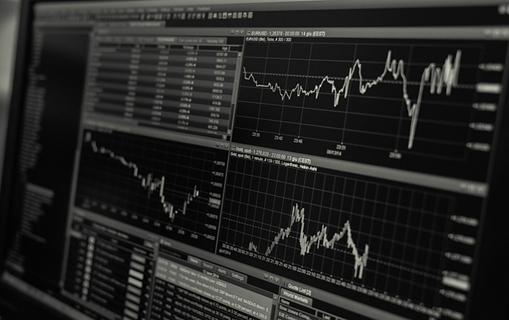
With the financial world evolving and recognizing the potential of machine learning, it becomes imperative for industry professionals to prepare for a future driven by machine learning.
Here are steps that individuals and organizations can take to embrace this transformative technology:
Building Machine Learning Expertise
Developing machine learning expertise is critical for creating and implementing these systems in finance. Expertise in machine learning can lead to numerous advantages, such as:
- Improved data analysis and speed
- Increased accuracy and efficiency
- Adaptive learning and continuous improvement
- Expanding investment opportunities
- Real-time market insights
- Risk management
- Unstructured data analysis
By investing in the development of machine learning expertise, financial professionals can better understand the potential benefits and challenges associated with it and ensure that they are fully prepared to harness its power in a rapidly evolving financial landscape. This can ultimately lead to more effective investment strategies and increased profitability in the world of finance.
Embracing Collaboration and Innovation
Collaboration between financial experts, data scientists, and technologists is essential to drive innovation and maximize the potential of machine learning in finance. By fostering a culture of collaboration and innovation, organizations can pool their resources, share knowledge, and generate novel ideas and solutions to address the unique challenges posed by machine learning in finance.
Nick Battaglia of Iowa explains that embracing collaboration and innovation can also help financial professionals stay ahead of the curve and capitalize on emerging opportunities in the market. By working together and embracing new ideas, professionals can ensure that they are fully prepared for a machine learning-driven future in finance.
Summary
In conclusion, machine learning has the potential to revolutionize the financial world and reshape the way investment strategies are devised and executed. By staying informed, cultivating machine learning expertise, and fostering a culture of collaboration and innovation, financial professionals can not only navigate the challenges and limitations of this cutting-edge technology but also harness its power to stay ahead of the curve and maximize returns in an ever-changing financial landscape.